The Growing Importance of Data Provenance
Remember when it used to be an amusing conversation, ‘the amount of data in the world is growing at blah blah blah rate….’?
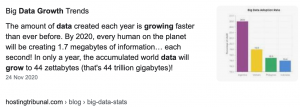
I would contend that whilst the base facts of these statements remain broadly true, that this is no longer so amusing. This huge growth in volume comes with baggage:
- It takes significant amounts of energy to gather and store all this data
- Gathering and storing data does not equate to use of that data; much of it is a replica of that already stored
- Terms and conditions in play at present across many scenarios place few limitations of onward sharing of data with other parties
- When volumes are huge and data movement so widespread and complex then keeping track of data provenance becomes very difficult
This post focuses on data provenance, which I think is one of the key disciplines required if we are to stay on top of and generate value from the huge array of information flows now in place. Whilst not in particularly common use in terminology, I would suggest that data provenance has been a key component in all data privacy/ protection regulations worldwide dating from the 1980’s; and it remains a key part of modern privacy regulations such as GDPR. Those familiar with privacy regulations will be well aware that the core of these regulations is to bring understanding and transparency around:
- What data types are in scope?
- Where are the data moving from?
- Where are they moving to?
- For what purpose are they moving?
- On what basis are they moving?
Answering those five questions is critical to compliance with privacy regulation; typically this has been done at an indicative / summary level rather than as a detailed line item listing. This summary model becomes very difficult to maintain and explain when the number, volume and complexity of data flows is growing so quickly. Indeed speaking from experience inside multiple large organisations; it is almost an impossibility for those responsible for privacy regulation compliance to maintain the answers to those five questions when they are not being done programmatically (i.e. via code rather than process).
Maintaining the answers to those five questions programmatically enables data provenance to be understood and maintained at scale. It also enables transition to/ adoption of data sharing agreements as an adjunct to a privacy policy, as recommended in the recent updated guidance on data sharing by the UK Information Commissioner. Data sharing agreements, according to this new guidance ‘set out the purpose of the data sharing, cover what happens to the data at each stage, set standards and help all the parties involved in sharing to be clear about their roles and responsibilities’. The benefits of the data sharing agreement approach are that they:
- aid transparency
- help all the parties be clear about their roles;
- set out the purpose of the data sharing;
- covers what happens to the data at each stage; and
- sets standards and expectations
That’s all well and good then, data sharing agreements are a step forward and now have official blessing. So let’s push that a bit further then with the concept of programmatic data sharing agreements; those underpinned by software code. In a subsequent post, I can cover how those can be built, for now I’ll set out what they enable. Consider if one could build:
- A comprehensive listing of data types recognised by a wide range of stakeholders, not least those representative of individuals (data subjects) who are the main beneficiaries of improved data sharing approaches
- A similarly comprehensive listing of data purposes, also recognised by a wide range of stakeholders, specifically including those representing individuals
- A comprehensive and accepted listing of legal bases under which personal data can be processed (which luckily and critically has already been delivered by GDPR)
Those first two are clearly quite a challenge, albeit such standardised listings have already begun to form in the shape of the standardised purpose lists within the IAB digital advertising framework.
Consider then the following proposal, first drafted by John Wunderlich and I at an IIW a couple of years back. The online spreadsheet below shows how, with the approach set out above, one can turn the build and deployment of data sharing agreements into a software supported process.
Combinations of Data Type being processed, data purposes, and legal bases for processing
Clearly this is only a first pass at the issue, but if one follows that logic then rather than privacy policies being opaque contracts with little chance of being read or understood by typical individual, data sharing agreements could become much more precise and thus transparent. Better still, the list of chosen set of data type x data purpose x legal basis permutations can be made machine readable along with any other relevant aspects of the data sharing agreement. When expressed in that way, it becomes much easier to make good practices accessible, or indeed poor practices more visible. In this sense, organisations who wish to demonstrate best practice in privacy, or who see privacy as a source of competitive advantage are the ones that should be progressing this way. And those who wish to continue to hide behind opaque privacy policies should avoid this direction for as long as they can.
You may ask what all this has to do with data provenance? Improved data provenance could be inferred but not guaranteed by the use of machine readable data sharing agreements as proposed above. The real improvement in data provenance emerges when the same standard list of data types, data purposes and legal bases for processing are used as tags/ metadata on all of the data flowing in the context of those data sharing agreements. That combination of capabilities delivers both contractual and technical support for high grade data provenance at the information flow level. Next post will be on data provenance at the individual attribute level using a Covid 19 example.