The visualisation below represents the data about themselves that an individual might wish to gather and use if they had the tools and the motivation to do so. So, ‘my data‘, for me and for my purposes; not the more typical ‘data on me’ gathered and used by many other parties.
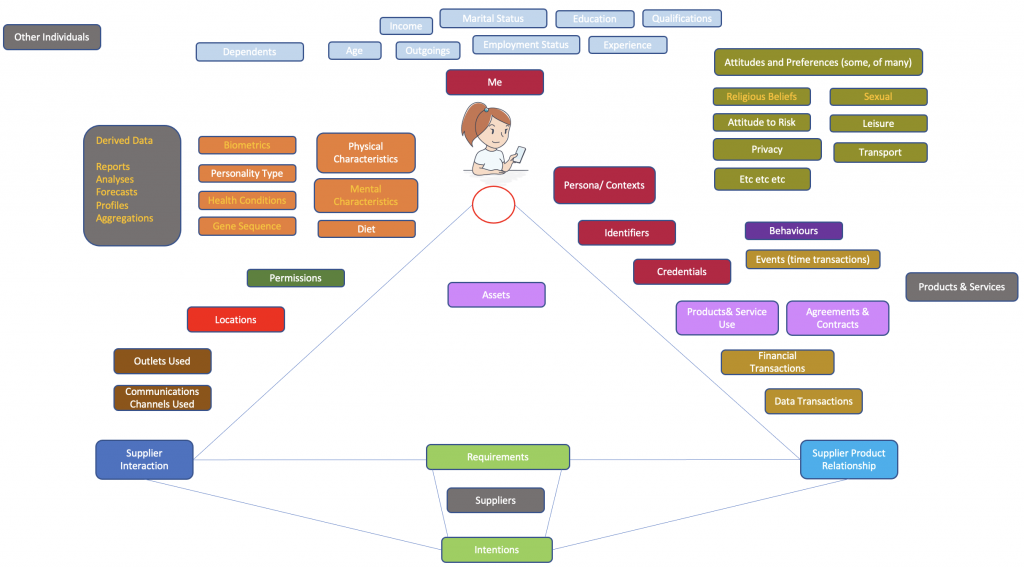
The key data types are described in more detail below. The colours in the diagram shows the higher level categories that i’m using to cluster these data types. And the orange text in the descriptions signify those data types that would typically be seen as sensitive data (in the legally defined sense).
Me: Whilst not a data type in its own right, this is required to anchor the diagram in a human-centric way.
Physical Characteristics: Those data attributes relating to the individual’s body.
Mental Characteristics: Those data attributes relating to the individual’s mind.
Diet: Those data attributes relating to the individual’s diet; i.e. intake of food and liquid.
Biometrics: Those data attributes relating to the individual’s body that are unique, and thus able to be utilised in identification processes and identity management (e.g. fingerprint, iris scan).
Personality Type: Those data attributes relating to the individual’s personality; often grouped into one or more aggregations/ categories that have evolved as science investigates the subject.
Health Conditions: Those data attributes and aggregations that define and help diagnose and manage conditions which are visible through measurement (i.e. generate data).
Gene Sequence: Those data attributes that can be derived when an individual undergoes a DNA sequencing process.
Marital Status: Typically a relatively simple status code and associated dates, locations and certifications.
Education: Those data attributes that are generated through formal education.
Qualifications: Typically a build on top of educational data, and extend into adulthood and the workplace.
Incomes: Typically an aggregation and derived from assets and financial transactions as a feed into the critical ‘net worth’, ‘disposable income’ and savings calculations.
Outgoings: Typically an aggregation and derived from assets and financial transactions as the other feed into the critical ‘net worth’, disposable income’ and savings calculations.
Employment Status: Typically a relatively simple status code, organisations, roles and associated dates, locations and certifications.
Experience: Often building out from education and employment, extending to cover softer data such as levels and types of experience.
Age: Simplistically this is just a birth date and number of years, months and days; but more complex/ detailed variants are also useful in some scenarios (e.g. biological age versus mental age).
Dependents: This is includes data about children, other family members; and opens up into the whole carer/ care recipient data set.
Persona/ Contexts: A human being typically operates in multiple roles some of which can be expressed digitally – for example, daughter, brother, mother, head of household, employee. Different persona require some additional data types appended to the core ‘me’.
Identifiers: These structured digital combinations of letters, numbers and characters are key parts of the digital plumbing we use on a day to day basis. Historically these digital identifiers have been created and issued by organisations to their customers, members and citizens. Critically when looking from the ‘my data’ perspective, individuals in their various roles/ personas/ contexts can generate their own identifiers. These will most likely be decentralised identifiers, as worked on here; the individual can generate and manage as many identities as they wish; one for each digital relationship would seem a good aspiration to prevent data leakage; subject obviously to managing this not becoming a huge challenge in its own right. Identifiers brought to the party by the individual are CRITICAL when it comes to re-balancing the relationship, and specifically the data sharing relationship, between individuals and the organisations they engage with.
Credentials: Are analogue or digital proofs; e.g. a passport, a driving licence, a tax code, a student entitlement card. Increasingly over time these can be delivered digitally, and typically build on top of the decentralised identifiers noted above. When my data is running at scale, individuals can draw in and utilise data from across a huge array of organisational relationships, and the data generated by them. At this point it becomes far more efficient to have the individual generate and manage their digital credentials and proofs, and we move much closer to the point where we can genuinely say that individuals are the optimal point of integration and generation of data about themselves.
Products: As a precursor to Product/ Service Use, and specifically NOT my data. Product (and Service) data on its own are the domain of the manufacturer and the supply chain; they are the ultimate experts in and describers of that which they bring to market.
Product/ Service Use: is where the individual and a product/ service join. The product is no longer the generic, it is the specific instance. Many products and services are not digital, and have no related data. But increasingly analogue products have digital components and thus a related data trail that the individuals can gather and curate. Nowadays, more and more products are actually online, wholly digital services. In those cases, the entire customer journey from onboarding through use and ultimately exit are digital, and thus generate data trails.
Agreements and Contracts: The data or indeed the signed documents that go alongside product/ service use. This includes the many hundreds of ‘contracts of adhesion’ that we all sign over the years.
Events (Time Transactions): Data that would typically be in a calendar, or somehow driven by time windows (e.g. i’m in the market for a new car between now and end of the current month).
Financial Transaction: Money in and money out of a financial services account of some type is the core of this type of data. Quality is often poor and ‘bank-centric’; open banking is designed to free up and improve this data type over time.
Data Transaction: Whilst far less obvious and understood than financial transactions, these are the lifeblood of The Internet, and the raw material for the well known ongoing exponential growth in data volumes. Each creation, read, update, deletion or aggregation of a data record creates a data transaction. In many cases they are not logged in any auditable, identifiable format, which leads to a chronic lack of data provenance in todays information systems.
Suppliers: Supplier data is typically in one sense outside of the scope of my data; until such time as we move from the generic supplier record which applies equally well to all parties, to the more specific ‘my supplier of’ record which taps into/ builds on the aforementioned core supplier record. Brand data is similar and seen alongside supplier data, although there is not a one to one alignment between the two. For example, Procter and Gamble is a supplier; but they run many brands, e.g. Ariel.
Supplier-Product Relationship: As with core supplier data, this data can begin outside the scope of my data when it remains generic (e.g. Ford make Mustangs) to the specific (Ford made my Mustang).
Requirements: This complex data type likely warrants a whole drill down blog post in its own right. It is the synthesis of individual and group needs and wants, expressed in data form. Ultimately these build out more from the Maslow root level, and are not just a simple list of things one is interested in buying or signing up for. In many cases requirements drives intentions, or intent data – the holy grail for marketers, and the primary bargaining chip the individual and the collective bring to the table in order to move beyond surveillance capitalism.
Assets: Another data type that likely needs its own page. Where ‘requirements’ amount to ‘what i’m in the market for’, assets equate to ‘what i’ve got already. There are many relationships between the two. That said, not all things that are bought/ got (financial or data transactions) become an asset. I’m not going to log a tube of toothpaste for example (although may do as a recurring requirement). But i’d expect to log the larger, higher impact things I have or have bought over and above that done with the base level product/ service use data. There are further complications around asset data caused by gifting and onward sale/ disposal; the individual is better placed than anyone to ensure accuracy of this data.
Supplier Interaction: This data type is the inbound and outbound interaction records between an individual and their existing and potential suppliers. Typically this would include data, the various parties concerned, the subject matter and any outcome.
Communications Channels Used: These would be the communications channels used by the individual, and the end point identifiers used (e.g. email addresses). It is reasonable to assume that over time these would also include the data API’s the individual uses to move data in and out of their data stores.
Outlets Used: Similar to supplier data, but this relates to specific outlets (physical or digital) through which a supply relationship interacts and transacts.
Behaviours: Behavioural data, often ‘observed’ from afar by suppliers and intermediaries working on their behalf is usually seen as something only relevant to those organisation doing the tracking and using the data. But this same data, or variants thereof, can also be used to help individuals better understand and ‘nudge’ their own behaviours, so moving this data to being under the control of individuals is a valid concept.
Locations: A high impact data type, includes every location that the individual deems to be important enough to gather data on. My home address, my work address, where I am right now; where I will be in 30 mins are all important examples. The delta between two locations is also important, not least to drive location to location information services.
Permissions: From the individual perspective, many complexities they face in online activity can be simplified into permissions; which can often be expressed as simple on/ off switches. This runs counter to the typical current model run by organisations which is to mask complicated requests and things they might not like could they see them behind long and obtuse terms and conditions and privacy policies. Ironically this lack of transparency from organisations is what led to GDPR, which in turn makes what could be managed as simple on/ off switches much more complex (6 authorities for processing for each data type for example).
Attitudes and Preferences: This broad and deep category of data types is perhaps the most difficult to pin down. Those listed below are
Religious Beliefs: This data type is almost always regarded as sensitive, as it should be given the possibilities of mis-use.
Sexual Preferences: Likewise, this data type is almost always regarded as sensitive, as it should be given the possibilities of mis-use. Clearly many applications/ businesses such as dating apps manage to look beyond that sensitivity to build viable business on this data type.
Attitude to Risk: This data type is key to sector such as wealth management, and most types of insurance. If this data type could be properly codified on the side of the individual then there are enormous possibilities for AI driven decision support tools.
Leisure Preferences: A straightforward set of choices on how one might spend leisure time; albeit the options list itself can be very large indeed.
Privacy Preferences: A more complex data-set, not least because of the lack of definition around the word ‘privacy’. In this section we are dealing with the larger, less tangible preferences; unlike the very specific ‘you cannot share my data outside of your organisation’ as would be found within the permission data type.
Transport Preferences: A straightforward list, at the top level at least, of preferred means of getting from A to B.
The above amounts to, in database terms, a listing of 3k to 3.5k data fields, depending on how far one wishes to push the boundaries of my data scope. There is no technical barrier to gathering, storing and using all of these data types FOR individuals rather than ON individuals. There are many norms and user experience reasons why that is not the case at present. The question therefore becomes what will be the drivers to gathering this data on the side of the individual. When that is so, this data-set will be hugely important and valuable to the individual, and managed accordingly.
Other Individuals: In data terms these act like Contacts in an Address Book, obviously each ‘contact’ has their own data store and can share as appropriate with others.